In the ever-evolving landscape of government fraud prevention, machine learning emerges as a powerful tool for identity authentication. This blog post delves into the intricate role of machine learning algorithms in identity authentication within government systems, exploring how anomaly detection, pattern recognition, and risk scoring models contribute to detecting suspicious behaviour and thwarting fraudulent activities.
Machine Learning in Identity Authentication
Machine learning algorithms leverage data patterns and statistical analysis to autonomously identify trends and anomalies. In the context of identity authentication, these algorithms play a crucial role in analysing vast datasets to detect irregularities and potential fraud indicators.
Anomaly Detection for Fraud Prevention
Anomaly detection algorithms excel at identifying deviations from established patterns or norms. In government systems, these algorithms scrutinise user behaviour, transactional data, and access patterns to flag unusual activities that may indicate fraudulent behaviour, such as unauthorized access attempts or unusual spending patterns.
Pattern Recognition for Enhanced Security
Pattern recognition algorithms analyse historical data to identify recurring patterns or trends. In identity authentication, these algorithms learn from past authentication attempts and user interactions to recognise legitimate behaviour and detect anomalies that may signal fraudulent activities, such as identity theft or account takeover attempts.
Risk Scoring Models for Predictive Analysis
Risk scoring models assess the likelihood of fraudulent activities based on various factors, including user behaviour, transaction history, and contextual information. By assigning risk scores to authentication attempts or transactions, these models enable government agencies to prioritise resources and take proactive measures to prevent fraud before it occurs.
Leveraging Machine Learning for Government Fraud Prevention
Government agencies can harness the power of machine learning in identity authentication through various applications:
- Real-time Fraud Detection: Machine learning algorithms continuously analyse incoming data streams to detect suspicious behaviour and trigger alerts for further investigation.
- Adaptive Authentication: Machine learning models adapt to evolving threats and user behaviour patterns, enhancing the accuracy of identity authentication while minimising false positives.
- Behavioural Biometrics: Machine learning algorithms analyse behavioural biometric traits, such as keystroke dynamics and mouse movements, to establish unique user profiles and detect anomalies indicative of fraudulent activities.
- Fraudulent Activity Prediction: Machine learning models forecast potential fraud risks based on historical data and emerging trends, enabling proactive fraud prevention measures and resource allocation.
Conclusion
Machine learning algorithms play a pivotal role in identity authentication within government systems, enabling proactive fraud detection and prevention through anomaly detection, pattern recognition, and risk scoring models. By leveraging the capabilities of machine learning, government agencies can enhance the security of their systems, safeguard sensitive data, and uphold the trust and confidence of citizens in their services.
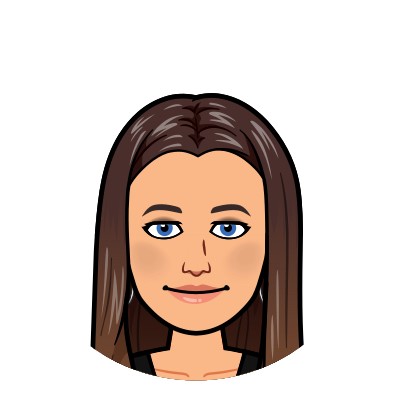
Nicole Lummis
After a career in Project Management, I realised how important it was for me to pursue a career with a more creative career path. I have a passion for creating engaging digital content whether through written content or the use of image, graphics or videos.