In the dynamic field of artificial intelligence, Natural Language Processing (NLP) modelling emerges as a revolutionary force, transforming how machines comprehend and generate human language. This comprehensive guide explores the intricacies of NLP modelling, investigating its applications, benefits, and best practices. Whether you're an experienced data scientist or an enthusiastic learner, join us on a journey to unravel the fascinating world of NLP modelling.
Understanding NLP Modelling
Defining NLP Modelling: NLP modelling involves crafting algorithms and computational models that empower machines to comprehend, interpret, and respond to human language. By leveraging machine learning techniques, these models aim to bridge the gap between human communication and artificial intelligence.
Key Components of NLP Modelling:
-
Tokenisation: The process of breaking down text into smaller units, or tokens, such as words or phrases. Tokenisation is a fundamental step in preparing text data for analysis and modelling.
-
Word Embeddings: Representing words as vectors in a multi-dimensional space. This technique captures semantic relationships between words, enabling the model to understand context and meaning.
-
Named Entity Recognition (NER): Identifying and classifying entities (such as names of people, organisations, and locations) within text. NER enhances the model's ability to extract valuable information.
-
Part-of-Speech Tagging: Assigning grammatical categories to words in a sentence. This helps the model understand the syntactic structure of language, contributing to more accurate analysis.
Applications of NLP Modelling
-
Sentiment Analysis: NLP models excel in gauging the sentiment expressed in textual data. Businesses use sentiment analysis to understand customer opinions, reviews, and feedback, informing strategic decision-making.
-
Chatbots and Virtual Assistants: NLP powers the conversational abilities of chatbots and virtual assistants. These applications can understand user queries, provide relevant information, and engage in natural language conversations.
-
Language Translation: NLP models are instrumental in language translation services. They can translate text from one language to another while preserving the intended meaning and context.
-
Text Summarisation: Automatic summarisation using NLP models condenses lengthy pieces of text into concise summaries. This is valuable for quickly extracting key information from large volumes of content.
-
Information Extraction: NLP facilitates the extraction of structured information from unstructured text. This is particularly useful in scenarios where valuable data is embedded in large amounts of textual content.
Benefits of NLP Modelling
-
Enhanced Efficiency: NLP models automate language-related tasks, saving time and resources. They can process and analyse vast amounts of textual data at speeds unattainable through manual efforts.
-
Improved Decision-Making: Businesses leverage NLP for data-driven decision-making. By extracting valuable insights from textual data, organisations can make informed choices and stay ahead in competitive landscapes.
-
Personalisation: NLP powers personalisation in user experiences. From tailored recommendations to customised responses, NLP models enable businesses to engage with users on an individual level.
-
Insights from Unstructured Data: Much of the world's data is unstructured, residing in text documents, emails, and social media. NLP models unlock insights from this unstructured data, providing a comprehensive view of information.
Best Practices for NLP Modelling
-
Quality Data is Key: NLP models heavily depend on the quality of training data. Ensure that your datasets are well-prepared, free from biases, and representative of the language diversity you aim to address.
-
Continuous Model Training: Language evolves, and so should your models. Regularly retrain NLP models with updated datasets to ensure they stay relevant and accurate over time.
-
Consideration of Context: Understanding context is crucial for NLP models. Consider the broader context in which language is used to avoid misinterpretations and improve the accuracy of predictions.
-
Ethical Considerations: Be mindful of ethical considerations, especially when dealing with sensitive topics. Ensure that your NLP models are designed and trained responsibly to avoid perpetuating biases or causing harm.
NLP Modelling - What's Coming Up...
As technology continues to advance, the future of NLP modelling holds exciting possibilities. The integration of advanced neural network architectures and the development of more context-aware models will likely shape the next generation of NLP applications. The evolution of multilingual models and increased support for low-resource languages will also contribute to a more inclusive and globally accessible digital landscape.
Conclusion
In conclusion, NLP modelling stands at the forefront of innovation, empowering machines to understand and interact with human language in unprecedented ways. From improving efficiency and decision-making to enabling personalised user experiences, the applications of NLP are vast and continually expanding. As you embark on your journey into the realm of NLP modelling, remember to stay abreast of advancements, adhere to best practices, and explore the limitless possibilities that natural language processing brings to the forefront of artificial intelligence. The road ahead is marked by endless opportunities to transform the way we communicate with and through machines.
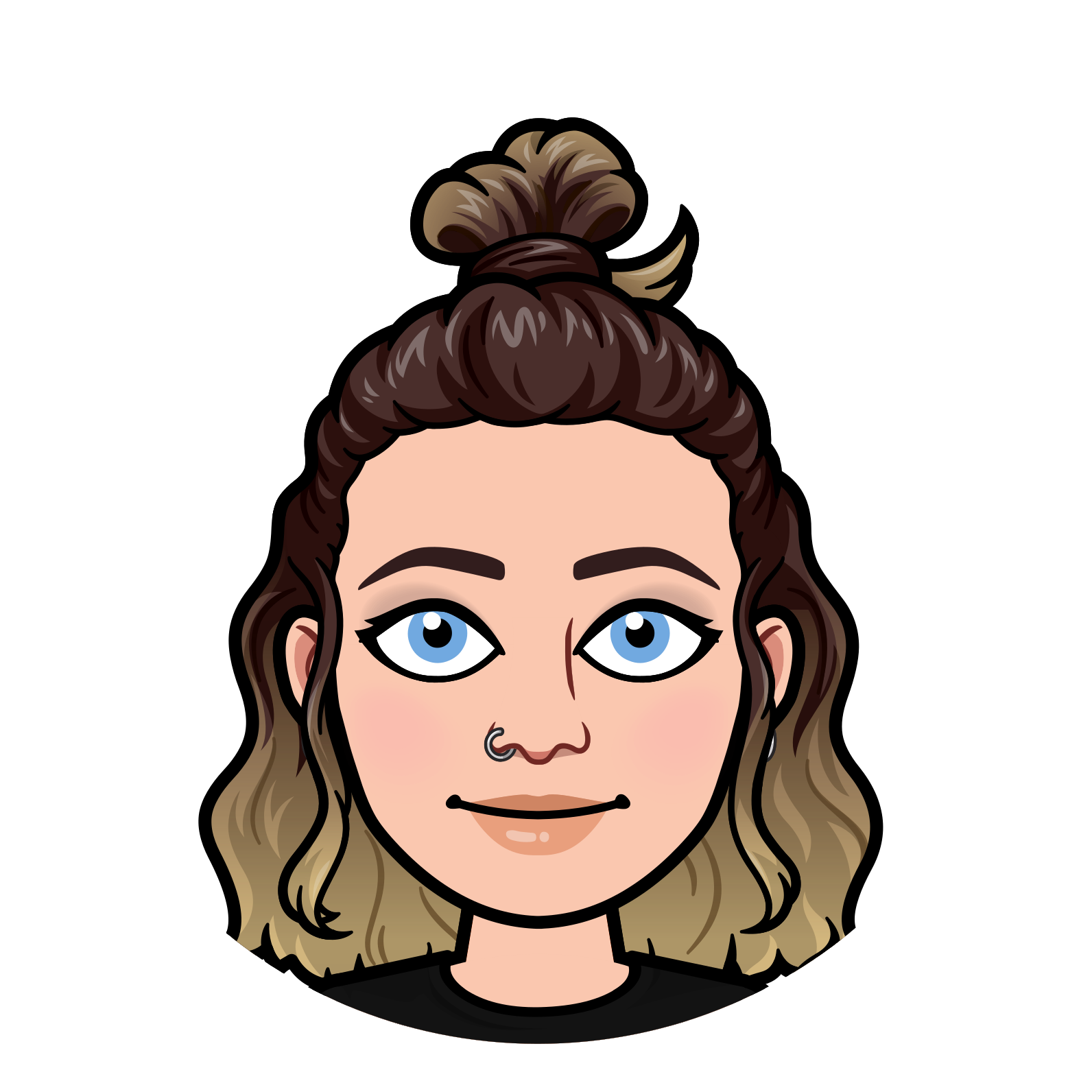
Julia Esgate Christmas
Experienced marketer with a demonstrated history of working in the events services industry. Marketing professional with a Bachelor of Arts (BA Hons) in English Literature from University of Brighton.