In the ever-evolving landscape of technology, data science stands as a driving force, revolutionising how businesses derive insights and make informed decisions. In this blog post, we'll delve into the realm of data science techniques, exploring their significance, applications, and the impact they have on shaping the future of industries.
Understanding Data Science Techniques
Data science techniques encompass a diverse set of methods and tools designed to extract valuable insights from large and complex datasets. These techniques leverage a combination of statistical analysis, machine learning algorithms, and domain expertise to uncover patterns, trends, and correlations within the data.
Significance of Data Science Techniques
-
Informed Decision-Making: Data science techniques empower businesses to make informed decisions based on evidence and analysis. By extracting meaningful insights from data, organisations can gain a competitive edge, identify market trends, and adapt strategies to changing conditions.
-
Predictive Analytics: One of the key applications of data science techniques is predictive analytics. By analysing historical data, businesses can predict future trends, customer behaviour, and market dynamics, enabling proactive decision-making and strategic planning.
-
Optimising Processes: Data science techniques play a crucial role in process optimisation. Whether it's streamlining supply chain operations, enhancing production processes, or optimising marketing campaigns, data-driven insights enable organisations to identify areas for improvement and efficiency.
Key Data Science Techniques
-
Machine Learning: At the forefront of data science techniques is machine learning. This subset of artificial intelligence involves the development of algorithms that allow systems to learn and improve from experience. Supervised learning, unsupervised learning, and reinforcement learning are common approaches within machine learning.
-
Statistical Analysis: Statistical analysis forms the foundation of many data science techniques. Descriptive statistics, inferential statistics, and hypothesis testing enable data scientists to draw meaningful conclusions and make predictions with a certain level of confidence.
-
Data Mining: Data mining involves uncovering patterns and relationships within large datasets. It includes techniques such as clustering, association rule mining, and anomaly detection, providing valuable insights into hidden trends and connections.
-
Natural Language Processing (NLP): NLP is a branch of artificial intelligence that focuses on the interaction between computers and human language. Sentiment analysis, text summarisation, and language translation are applications of NLP within data science, unlocking valuable information from unstructured text data.
Implementing Data Science Techniques
-
Data Collection and Cleaning: The first step in implementing data science techniques is collecting relevant data. Once obtained, the data must be cleaned and preprocessed to ensure accuracy and remove any inconsistencies that could impact analysis.
-
Exploratory Data Analysis (EDA): EDA involves visually exploring and summarising datasets to gain a preliminary understanding of the underlying patterns. Techniques such as data visualisation, summary statistics, and correlation analysis are employed during this phase.
-
Model Building and Training: For machine learning applications, data scientists build and train models using algorithms suitable for the task at hand. This involves splitting the dataset into training and testing sets, fine-tuning parameters, and assessing model performance.
-
Evaluation and Iteration: After deploying a model, it's crucial to evaluate its performance against real-world data. Data scientists iterate on the model, making adjustments as needed to enhance accuracy and adapt to changing conditions.
Challenges in Data Science Techniques
-
Data Quality: The accuracy and reliability of insights derived from data science techniques heavily depend on the quality of the input data. Inaccuracies, inconsistencies, and missing values can compromise the validity of results.
-
Interpretability: Some advanced machine learning models, such as neural networks, are often seen as "black boxes," making it challenging to interpret how they arrive at specific conclusions. Ensuring model interpretability remains a critical challenge in the field.
The Future of Data Science Techniques
As technology advances, the landscape of data science continues to evolve. The integration of artificial intelligence, the expansion of edge computing, and the increasing availability of big data are reshaping the possibilities within the field. Continued research and innovation in data science techniques promise to unlock new avenues for exploration and discovery.
In conclusion, data science techniques are at the forefront of innovation, driving businesses towards a future where insights derived from data pave the way for success. From predictive analytics to machine learning, these techniques empower organisations to make informed decisions, optimise processes, and stay ahead in a rapidly changing digital landscape. As the world becomes increasingly data-driven, mastering these techniques is not just a choice but a necessity for businesses seeking to thrive in the modern era.
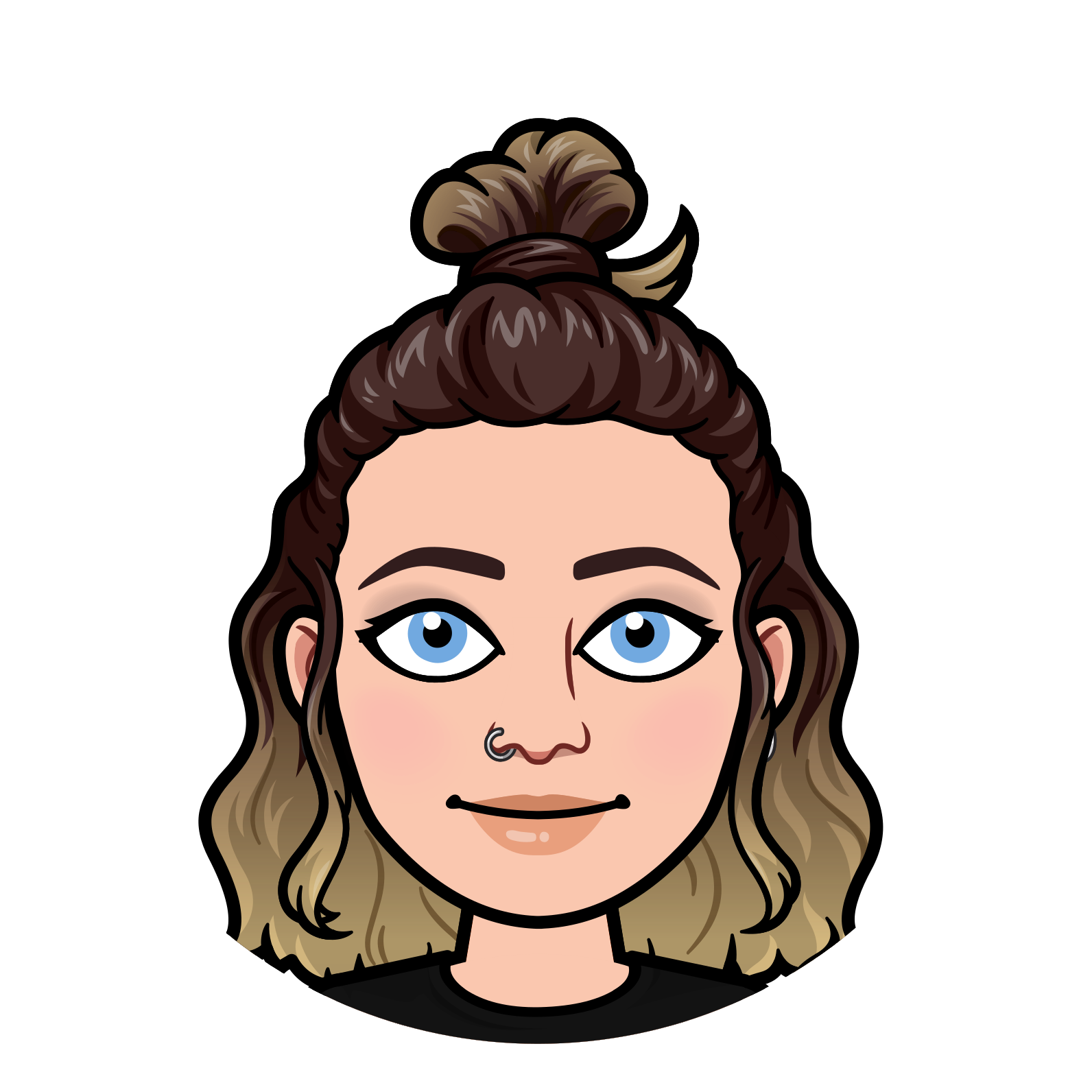
Julia Esgate Christmas
Experienced marketer with a demonstrated history of working in the events services industry. Marketing professional with a Bachelor of Arts (BA Hons) in English Literature from University of Brighton.